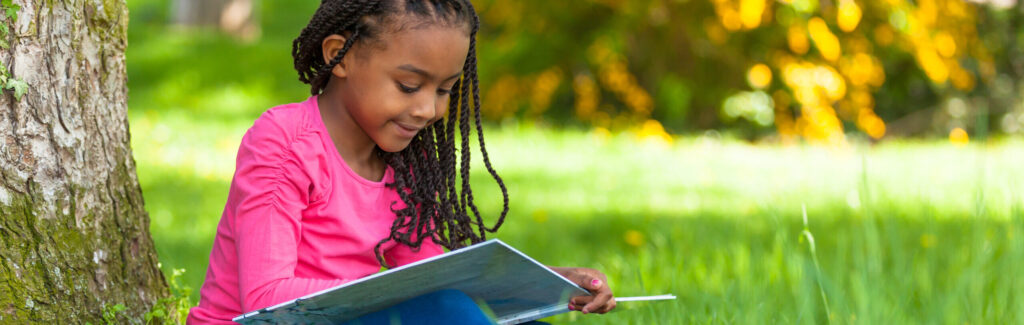
How gamification is changing education
Out and about in town, it’s not unusual to see Fortnite t-shirts and lunch boxes in shop windows, joggers feverishly
Out and about in town, it’s not unusual to see Fortnite t-shirts and lunch boxes in shop windows, joggers feverishly
Book a chat with our team
If you’d like to use Doodle’s browser version, please visit this page on a desktop.
To log in to Doodle on this device, you can do so through our apps. You can find out how to download them here: